In part 1 we saw how my candid curiosity got me into a Twitter fight of epic proportions. While unpleasant, it did have the positive side-effect of bringing actual experts into the fray, which helped dispel some of my misconceptions, and clarified the reasons for my own lack of enthusiasm for RNA-based COVID vaccines (the only ones currently available on the US market).
The premise for my questioning was that, while many proponents of COVID vaccination take it as an article of faith that it is the best way out of this mess, the case was less clear to me, and I (admittedly awkwardly) solicited the hive mind’s input on three questions:
Question 1: Do vaccines help reduce asymptomatic transmission?
Early in the pandemic, I had heard that asymptomatic transmission accounted for nearly 85% of infections, which is why I was so curious as to what vaccines do to such transmission. It was abundantly clear from clinical trials that vaccines protect individuals from developing severe forms of the illness, but if that didn’t translate into reduced transmission, I could not see how that alone was going to help us end the pandemic. Sure, there is the argument that reducing viral load overall should reduce transmission, as expressed, for instance, in Tara Smith’s point #6:
Yet, working with climate models, I’m well acquainted with things that should work in theory, but don’t always, in practice, so it’s always a good idea to test before we trust. This is why I was so eager to see data on this, and lo and behold, Tara pointed to a very recent article which suggests:
previous receipt of an mRNA COVID-19 vaccine was associated with an 80% reduction of risk in asymptomatic COVID-19 in patients that have received 2 vaccines when compared to those who had not been vaccinated.
source: Tande et al, 2021
So yes, the mRNA-based vaccines used in the study (the Pfizer and Moderna ones) do appear to reduce transmission. That’s all was I asking for. In case you thought that was obvious, I hope you will grant me that there are worse crimes than not being aware of a paper that was published 5 days prior to consulting the hive mind. I doubt most of people screaming at me were even aware of it.
Perhaps they knew it did not matter so much? It appears that I was confusing two distinct concepts: pre-symptomatic vs symptomless transmission, though this is partly – I was told – because the two are often lumped together even by competent experts. Pre-symptomatic transmission is related to the fact that viral loads, and therefore contagiousness, appear to be highest in the few days prior to developing symptoms. Distinct from that, many individuals never develop any detectable symptoms, yet are able to infect others (“symptomless” transmission). It is that latter mode that I thought was responsible for 85% of transmission, but, in the words of a recent perspective in Science:
The prevalence of symptomless cases is not precisely established. Early studies reported that asymptomatic cases accounted for 30 to 80% of infections, but more recent data point to a rate of asymptomatic cases between 17 and 30%.
Rasmussen & Popescu, 2021
They go on to say:
Determining the true transmission capability of asymptomatic and presymptomatic cases is inherently complex, but knowledge gaps should not detract from acknowledging their role in the spread of SARS-CoV-2. [….] [E]mphasis on the degree of contagiousness rather than the knowledge that people without symptoms are generally contagious detracts from the public health threat that asymptomatic and presymptomatic infections pose and the need for continuous community-based surveillance and interventions.
Rasmussen & Popescu, 2021
The figure below, from the same source, qualitatively describes what is presently known about this:
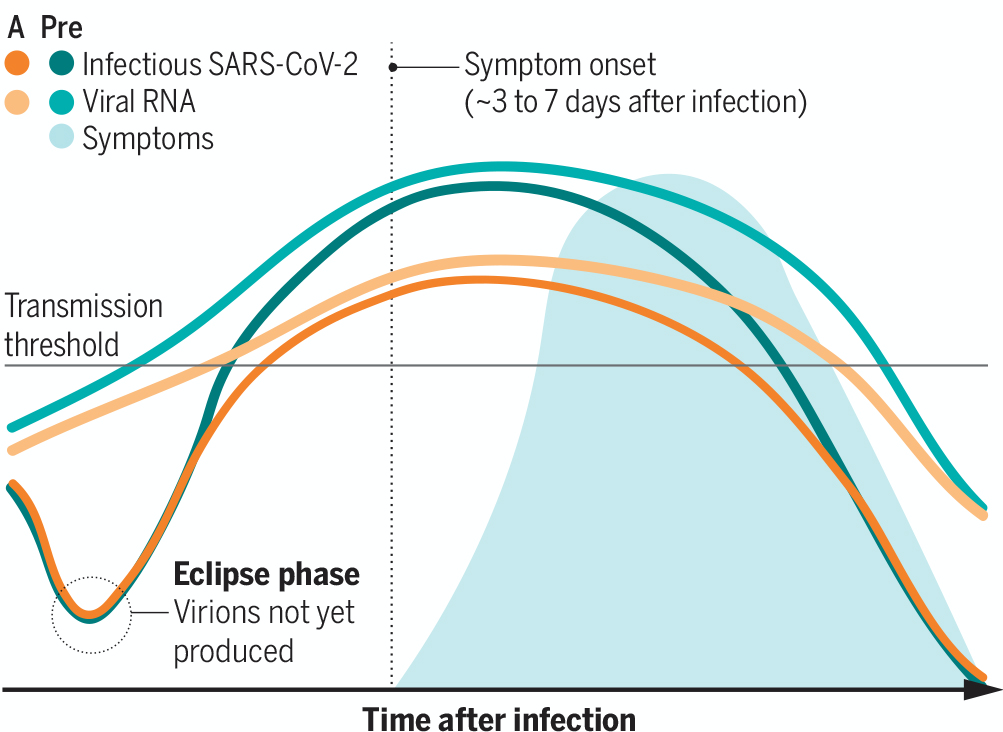
To me, what this means is that we will need to continue testing regularly to see if interventions (including vaccinations) are working. Indeed, if mass vaccination is successful, the end result will be that the overwhelming majority of residual transmission is carried out by individuals that exhibit weak to zero symptoms. Recent data make it clear that the two COVID vaccines presently used in the US should markedly help with reducing asymptomatic transmission. This fact wasn’t obvious to me last week (nor when Tara Smith wrote this piece), but now it is. Thank you, experts. Vaccines 1, hesitancy 0.
Question 2: Are the COVID-19 vaccines safe?
Here I got a little too quickly assimilated to a garden-variety anti-vaxxer, so let me make one thing clear. You can be 100% convinced of the thoroughly well-founded nature of vaccination while wanting more information on COVID vaccines. The reason is that mRNA vaccines are a very novel beast, so the sleight of hand that “vaccines are safe” (which, again, is true in general) does not imply that this is automatically the case here. To illustrate this, consider that Moderna had prior to COVID-19 been in the business of making such vaccines for about a decade and that the COVID vaccine is the first one that got approved by the FDA (under an Emergency Use Authorization, need I say). Many expect that COVID is the watershed moment for this technology; by allowing lightning fast design (Moderna started testing theirs a week after the wild COVID genome had been sequenced), it establishes the technology as uniquely suited to responding to rapidly-emerging pathogens and their mutations. Some expect it will durably change vaccine design, enabling flu shots to (finally) be responsive to whatever flu strain actually hits our shores, not the one models predict might emerge 6 months later. And yes, even mRNA vaccine enthusiasts will admit that the lipid nanoparticles that act as vectors for the genetic material have problematic side effects.
It’s therefore not entirely unreasonable to ask if those vaccines are safe. Naturally, the clinical trials were designed to test for that, and it’s remarkable that a vaccine developed in such a short time was able to clear those hurdles. But it is this very swiftness that gives some people pause about the vaccines. Yet, Tara Haelle argues that, given that one year of data has now accumulated:
If the Covid-19 vaccine caused long-term effects beyond the side effects reported in the trials, we would know about them by now.
Tara Haelle
This is where I must admit to being more conservative. One year is hardly an eternity, and indeed, the reason the vaccines are not being mandated right now is that, under FDA rules, you’d need at least 3 years of data to establish long-term safety. Moreover, there are documented instances of side effects that take longer to detect: the deadly history of Vioxx, which only surfaced after about 5 years on the market, comes to mind. So one could be forgiven for wondering whether one year really is enough to establish safety.
So how should one rationally evaluate a fundamentally new vaccine? Let us not indulge in our innate tendency for zero-risk bias. The house is on fire, and before we can think about redesigning the kitchen, we have to put out the fire. These mRNA vaccines appear to be our best shot at doing so, so this is clearly the lesser-evil.
Vaccines 2, hesitancy 0.
Question 3: how effective is the protection?
We now come to my third question: are the current vaccines the best way to quell the infection in the long-term? I had two concerns here:
- Re-infections are possible, casting doubt on the long-term protection provided by COVID antibodies, even if the virus is a fixed target.
- What’s more, the virus is very much a moving target. Some variants (most notably the one known as B.1.351, first identified in South Africa) are now accounting for 20-30% of new infections in the US, and are resistant to the Pfizer and Moderna vaccines (the latter, thankfully, work on variant B.1.1.7).
Leaving aside issues of access and distribution, it is hard to envision how, even under the most ideal of circumstances (e.g. scoundrels like myself impaling themselves onto the first vaccine-laden syringe in sight), vaccines could bring about the much sought-after herd immunity. Unlike measles, where the pathogen is essentially fixed and antibody protection lasts for years, fighting this Learnean hydra with a single weapon seems a losing battle, and I wanted reassurance that there was a more cunning plan in the works.
Zeynep Tufekci writes:
The solution is obvious and doable: We should immediately match variant surges with vaccination surges that target the most vulnerable by going where they are, in the cities and states experiencing active outbreaks—an effort modeled on a public-health tool called “ring vaccination.” Ring vaccination involves vaccinating contacts and potential contacts of cases, essentially smothering the outbreak by surrounding it with immunity. We should do this, but on a surge scale, essentially ring-vaccinating whole cities and even states.
That makes a lot of sense to me, and this is something I can get excited about. Indeed, I suggested something similar, and got called all kinds of names for it.
Last month, Moderna shipped a cocktail of such vaccines to the NIH for clinical study. It’s unclear how large those trials will be, though if there has been one silver lining with the calamitous handling of the pandemic by the Trump administration, it is that there are no shortage of individuals and opportunities for infections to conduct large clinical trials. I can imagine that, as we learn about these new variants and as models help forecast their diffusion in the population, we might be able to continuously adjust the vaccine to keep hitting this moving target. This appears a significant advantage of mRNA-based vaccines over traditional, protein-subunit vaccines; the infrastructure is flexible enough that it allows to quickly load a “patch” to the operating system, and ensure that the vaccines do protect against the most relevant strains of the virus. Caveats about long-term safety still apply, but it seems fair to assume that the risk profile will be similar regardless of what exact RNA sequence is being included in the vector (as the side affects are thought to be related to the lipid nanoparticles that encapsulate those sequences).
Still, for this game to work, you’d have to vaccine all eligible individuals very regularly, to stamp out any opportunity for the virus to spread and mutate. This may be possible in the type of altruistic utopia I’ve previously written about, but the US and other “First World” nations have definitely taken a “Me First” approach to this (as usual), and until that changes, there will be unprotected populations where the virus is free to evolve. And somehow, even under the restrictive policies put in place in my home state of California, international travel was always a bigger priority than kids going to school, so we gave up long ago on containing the virus to any part of the world (the ethics of which are repugnant anyway). So while the vaccine seems like the best way to tame the fire in the US, it’s unclear to me whether it will truly extinguish it. That is why more knowledgeable people than me have been warning of a permanent pandemic. Much of this may be have been obvious to you long ago, so you may wonder why I am wasting precious time rehashing old news. As I hope to have illustrated through this partial set of examples, much of the news are still rather fresh; forgive me if it takes me a few weeks to adjust.
So where do we stand? With help from the Knowing Taras and some digging of my own, I am now 100% convinced that mRNA-based vaccines are our best bet at controlling the pandemic in the short term. But since I’m a long-term kind of guy (I study climate change over scales of years to millennia for a living), forgive me if I close these reflections with thoughts for the long-term.
Beyond Vaccines
I’d be setting up a strawman if I said that all vaccine enthusiasts see COVID vaccination as a necessary and sufficient condition to get back to “normal”, but I’ve definitely met people who do, and there are a few problems with that worldview. Below I outline a few reasons to be wary of a strategy that prioritizes vaccination at the exclusion of any other solution. Once again, please don’t paint me with the seal of anti-vaxxer infamy for saying that: I do believe vaccines are useful; I just don’t see them as the be-all-end-all that so many people desperately want them to be.
Normal wasn’t that great for everyone
To me, the great lesson of COVID-19 is one of inequality: the pandemic has magnified pre-existing health disparities, like diabetes or obesity, which largely map onto economic and environmental disparities, themselves mapping largely onto racial lines, with Latino, Back or Indigenous communities having suffered the greatest losses. These disparities won’t go away once 85%+ of the US population (including myself) gets vaccinated. Getting back to the pre-2020 norm is only an enticing prospect if that norm is remotely fair, which it never was: African Americans were being poisoned by lead in Flint, MI; kids in poor LA neighborhoods were at higher risk of asthma, the list goes on. To me, COVID’s co-morbidities call for a renewed focus on the social and environmental determinants of health, including access to clean water, clean air, clean food, exercise, and a holistic understanding of health. That isn’t quite the message sent by many companies promising free glazed donuts, beer, or popcorn for getting vaccinated. Yes, I too yearn for resuming social activities, but this sounds a lot like consumerism. And in case you haven’t noticed, COVID is associated with the very same co-morbidities as consumerism, namely obesity, type II diabetes, smoking, and others. Do we have a societal plan for truly addressing that?
No Silver Bullet
Given that not everyone can be vaccinated, vaccines are therefore a partial shield against infection (a useful one, for sure). Thus, it only makes sense as part of a broader strategy to truly address the root causes of COVID inequality (i.e. why some people get so much sicker than others). I’m sure some health practitioners see it that way as well, but that is conspicuously absent from the public conversation. This concerns me, because it risks setting up impossible expectations around vaccines that could backfire. And once again, I think vaccines are awesome, just not a substitute for healthy living.
To return to a climate analogy, a vaccine-only strategy against COVID is like a strategy against anthropogenic global warming that would rely on solar radiation management, say. In both cases, it is treating symptoms, using interventions that fail to address the root cause of the problem (in the case of climate change: carbon emissions), a techno-fix mediated by for-profit companies, allowing society to function largely as it did before, without any fundamental reorganization or rethinking. Treating symptoms is something our industrial societies are very good at doing, and I was hoping this pandemic would force us to focus more on underlying causes. Looks like we’re not quite there yet.
Health Fundamentals
The other great lesson of COVID, for me, is one of humility: yes, it is amazing that not one, but two 95% effective vaccine clearing phase 3 clinical trials was developed under 9 months. Yay science. But I also remain very curious about all the unknowns of this virus: why does it steamroll some seemingly healthy individuals while leaving others in comparable demographics completely unscathed? Why do some people recover quickly while some get mired in a “long COVID” nightmare? I don’t claim to have my finger on the pulse of that literature, but everything I have read suggests that we know very little about those fundamentals. SARS-CoV-2 has exposed some deep gaps in our understanding of the human body, and all the trumpets and fanfare about vaccine development (it’s cool, I get it) should not detract from the fact that our understanding of health is still worryingly incomplete. Going back to the climate analogy, it would be like trusting a model-based climate intervention based on models that can’t satisfactorily represent the modern-day climate.
This is more than an issue of modeling. It goes to the very core philosophy. Indeed, one of the central tenets of Western medicine is that:
all bodies respond roughly the same way to infection or injury, and the immune system is a well-organized defense mechanism that never attacks the body. […] The framework dates back to the embrace of germ theory in the late 19th century. The idea that many illnesses are caused by an observable pathogen, which produces distinct and predictable symptoms, had a dramatic clarity to it. It pushed Western medicine away from an earlier holistic emphasis on the role an individual’s constitution played in illness. According to the new view, infection was determined by a specific and measurable entity. Focus had shifted from the soil to the seed, as it were.
Meghan O’Rourke, Can Long COVID be treated?
This philosophy has been extraordinarily effective for some pathologies (like childhood infectious diseases), far more effective than competing systems like traditional Chinese medicine or Ayurvedic medicine. However, the mysteries of long COVID, and other ones, should be cause for a little humility. In the same article, Megan O’Rourke draws parallel between long COVID and other ill-understood pathologies that are often undiagnosed or written off, either associated with the long after-effects of viral infections (Epstein-Barr, influenza) or chronic conditions like myalgic encephalomyelitis/chronic fatigue syndrome. She writes:
many researchers I spoke with believe that the race to understand long COVID will advance our understanding of chronic conditions that follow infection, transforming medicine in the process.
Meghan O’Rourke, Can Long COVID be treated?
Few serious medical practitioners would dispute that there are a several things for which Western medicine has no good theory. Here are three that I believe pertinent to COVID19:
- the placebo effect (it’s undeniable, yet we can’t explain it)
- auto-immune diseases
- the innocuous common cold (some variants are which are caused by human coronaviruses).
To me, these are pretty fundamental things; I’d be wary of putting all my trust into a system that cannot account for that. If you think it makes me “anti-science” to acknowledge the current limits of Western science, then you’re part of why that understanding is limited. I have faith in the process of science to (eventually) reach more and more complete explanations of the world, but this can only happen if we are honest about knowledge gaps. I don’t have indiscriminate faith in all the solutions currently provided by allopathic medicine, especially when said solutions are so transparently tied to the profit motive. What’s the alternative, you say?
The Science of Breath
“Alternative” medicine is any medicine that Western medical doctors didn’t learn in school, much like “World music” is a Western moniker for any music that isn’t classical, jazz, rock and hip-hop, pretty much; that is to say, most music created by humankind. In the same article on “long COVID”, I was struck to read that some of the immune and cardiac symptoms experienced by patients were tied to dysautonomia, an “impairment of the usual functioning of the autonomic nervous system, which controls blood pressure, temperature regulation, and digestion. ” For thousands of years, these are processes that yogis have learned to control by manipulating one of our most essential biological functions, and one that a respiratory syndrome like SARS-CoV-2 attacks most violently: the breath. The logic for using the breath is deceptively simple: breathing is a largely autonomous function over which we have conscious control. It therefore provides a backdoor to the operating system of our body and mind – such is the central claim of pranayama, the science of breath. If you like to scorn ancient wisdom, be reassured: the book I just linked to was co-authored by two competent MDs.
Continuing the article, I therefore wasn’t too surprised to learn that some patients experienced dramatic improvements by practicing breathing exercises, in this case the Stasis program which “involves inhaling and exhaling through your nose in prescribed counts in the morning and at night.” The paper version of the article included a helpful visualization of this process, which the online version unfortunately left out. Though it is different in detail, it reminded me of a pranayama technique called kumbhaka, one variant of which is described here.
This would not be the first time that Westerners “discover” ancient knowledge: meditation used to be a woo-woo oddity reserved for Tibetan monks and Westerners who’ve lost their way. Now it’s all the rage in even the most conservative corporations. Yoga used to be a weird practice for pretzel-legged sadhus; now you can get your health insurance to subsidize your membership at the local studio (it helped my sciatica better than any drug could have). The list goes on. If the West has caught up, in just a few decades, on knowledge that Yogic and Tibetan scriptures have documented for thousands of years, there is hope! Perhaps in the next decade allopathic medicine will catch on to the importance of breath? If it took COVID to get there, maybe that will have been worth it. Either way, I practice various breathing techniques daily, and the Long Quarantine only further crystallized its importance in my life.
Before getting further misunderstood, let me make this plain: I’m not saying that this or that breathing technique will cure COVID. What I am saying is that the ancient knowledge of pranayama (and, more generally, the contemplative traditions of the East), which is periodically co-opted and repackaged by Western health practitioners (for a fee), can prove surprisingly effective where allopathic medicine fails miserably. It’s – at the very least – worth a shot, given the rather favorable risk profile of breathing (even intensely) compared to any imaginable pharmaceutical intervention. In the article, several patients reported that those breath manipulations had helped them like nothing else, though it is still a very long climb back up for many of them.
There are many more dimensions to building a health system that’s truly about Health, and not just Sickness. They will have to wait for another post (or rather, a true expert on the matter). I now want to address what I believe caused the strong allergic reaction to my original thread.
I don’t speak for the trees (or geoscientists)
My biggest fault (aside from unwisely rousing the Twitter monster with poorly considered words) lies in casting those words as “my Earth Science position on the matter.” To clarify, this has nothing to do with the Earth Sciences. I do not have the right to speak for my community on any topic, much less a topic in which I have not been trained. I believe that is why so many in my community took issue with the thread; had I not done this, I can only assume that the disagreement would have been voiced more politely. If you are one of those geoscientists who felt singularly misrepresented by my words, please receive my sincere apologies. I do not speak for geoscientists.
What this is about is the process of making decisions in the face of much uncertainty and urgency. As a climate scientist I have longed been exasperated that some people, after all this time, are still skeptical of the unequivocal human influence on climate. I have voiced that impatience many times, including on this blog. What this experience has taught me is that it is not overly pleasant being on the other side of that table: trying to make a rational decision about so consequential a topic in the midst of a health crisis is a recipe for cognitive biases (particularly the affect heuristic) to kick in, leaving minds particularly prone to bad decisions. What I was attempting was an experiment in self-awareness, a journey through the meanderings of decision-making, drawing on friends that I hoped as evidence-minded as I am to share with me the evidence that convinced them.
I can understand that those who had made up their mind long ago, perhaps drawing on more scholarship than I did, would find this process useless at best, or even overindulgent. Some went so far as to call it dangerous or harmful, which is vastly overstating my social media reach. Yet, I hope documenting this process will be of use for those who still find themselves hesitant to take the COVID vaccine plunge. An interesting lesson I drew from the (biased) sample of responders is that they were more royalist than the king: the default position appears to be “how dare you question experts?”, while the two actual experts that got dragged into the thread (the Knowing Taras) revealed themselves much more tolerant of my curiosity. No doubt sample size is insufficient to draw very reliable conclusions here; yet, it made me wonder why some of my fellow climate scientists were so quick to shut down anything remotely redolent of disbelieving an expert consensus.
After decades of being outmaneuvered by climate denialists in the court of public opinion, I can certainly sympathize with the sentiment of many climate scientists (“oh no, not again!”) and the knee-jerk reaction it inspired. Yet, what I learned through unwittingly turning the tables (suddenly becoming the questioning non-expert thrust in front of several sharp-tongued climate scientists) is that “trust experts and shut up” is a really ineffective form of science communication. It did not work on me, and it does not, apparently, work on many people. That is one reason why we are still struggling in the court of public opinion in communicating just how much how damn effing sure we are that human activities are warming the Earth. Beyond the diabolical efficacy of the Merchants of Doubt at obscuring this consensus, it is clear that the generalized mistrust of all manner of experts flies in the face of a “trust experts and shut up” communication strategy. I am not saying there is a universal answer, but starting from an assumption of good faith might not be the worst thing in the world.
Certainly the Knowing Taras engaged in a much more productive dialogue, one that will inspire me, in the future, to be an expert worth consulting without getting yelled at. I think we’ll all appear more trustworthy if we start there.
So, am I getting vaccinated?
Yes, indeed I am. I ‘d be lying if I said that I experience vaccine envy, for reasons mentioned above. But at this point it would be foolish to deny that the pros of vaccination so forcefully outweigh the cons. I’ll be getting vaccinated and I’ll post about it. But I’ll also keep taking care of my health and I hope you do the same. If I hadn’t quit drinking during this pandemic, I’d raise a glass to your good health.
Thank you for reading this far. (Civil) comments are welcome.